Picture 1 of 2
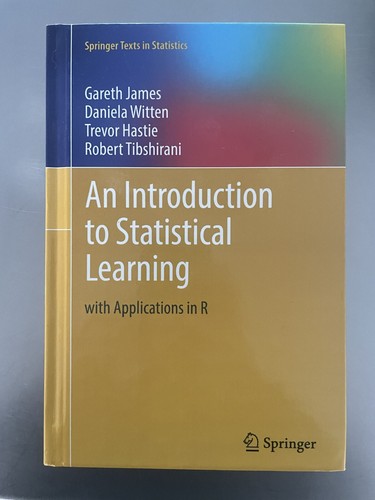
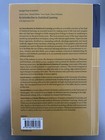
Gallery
Picture 1 of 2
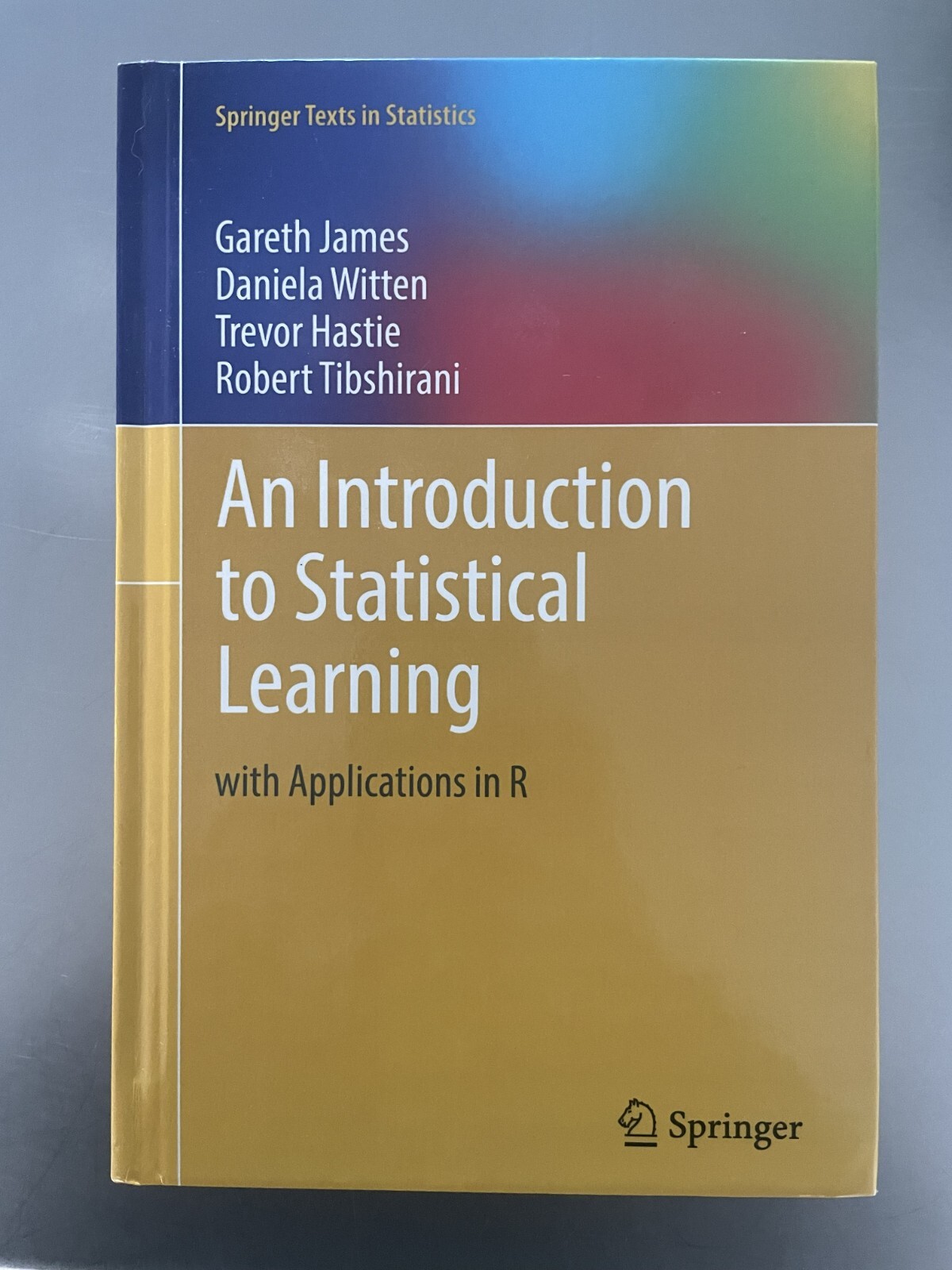
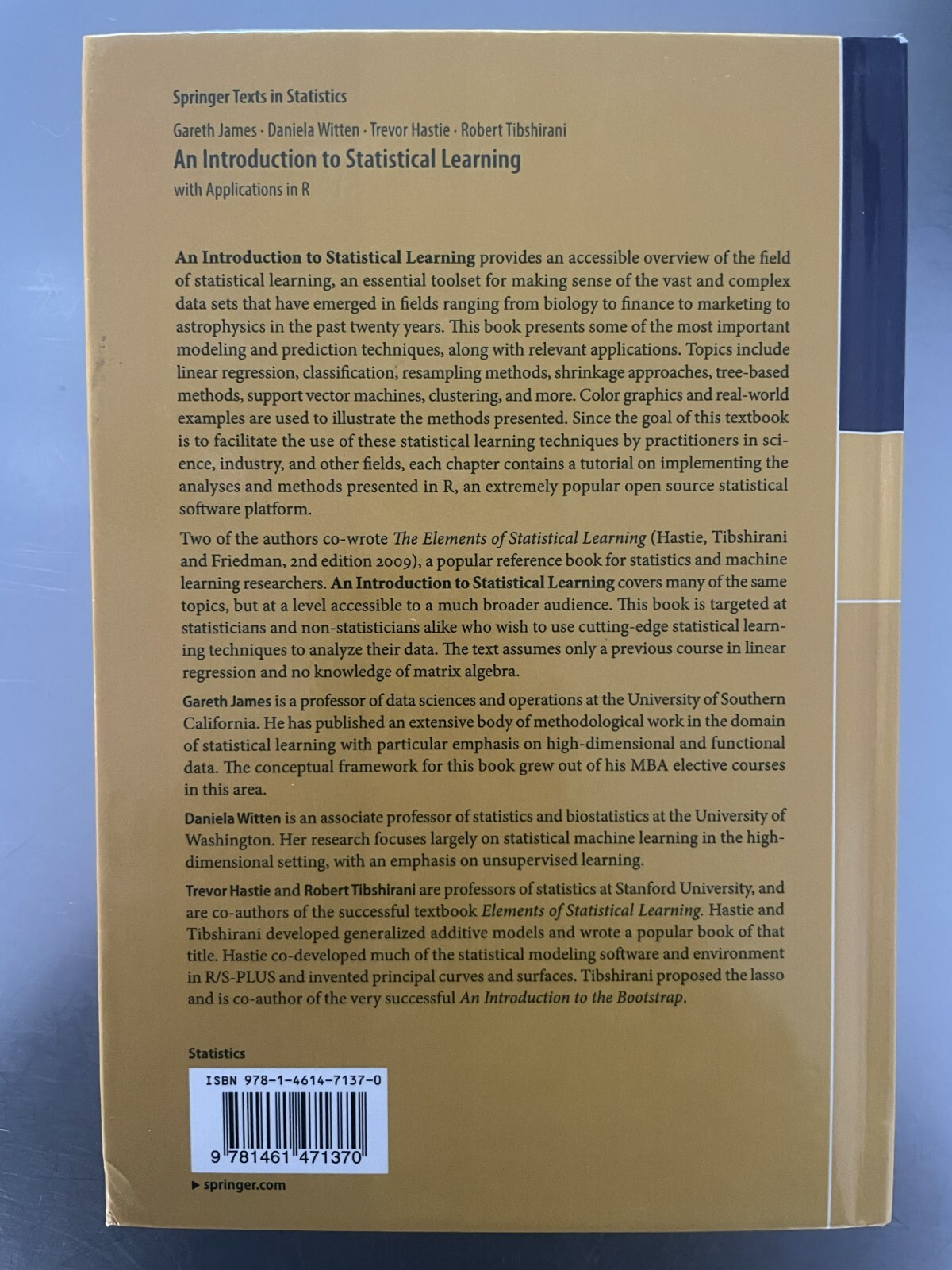
Springer Texts in Statistics Ser.: An Introduction to Statistical Learning :...
US $50.00
Approximately£37.72
or Best Offer
Condition:
New
A new, unread, unused book in perfect condition with no missing or damaged pages. See the seller's listing for full details.
Oops! Looks like we're having trouble connecting to our server.
Refresh your browser window to try again.
Postage:
US $5.38 (approx £4.06) USPS Media MailTM.
Located in: Chicago, Illinois, United States
Delivery:
Estimated between Mon, 28 Apr and Sat, 3 May to 43230
Returns:
No returns accepted.
Payments:
Shop with confidence
Seller assumes all responsibility for this listing.
eBay item number:285243417253
Item specifics
- Condition
- Subject
- Statistics
- ISBN
- 9781071614174
- EAN
- 9781071614174
About this product
Product Identifiers
Publisher
Springer
ISBN-10
1071614177
ISBN-13
9781071614174
eBay Product ID (ePID)
17050082535
Product Key Features
Number of Pages
Xv, 607 Pages
Language
English
Publication Name
Introduction to Statistical Learning : with Applications in R
Subject
Mathematical & Statistical Software, Probability & Statistics / General, Intelligence (Ai) & Semantics, General
Publication Year
2021
Type
Textbook
Subject Area
Mathematics, Computers
Series
Springer Texts in Statistics Ser.
Format
Hardcover
Dimensions
Item Weight
42 Oz
Item Length
9.3 in
Item Width
6.1 in
Additional Product Features
Edition Number
2
TitleLeading
An
Dewey Edition
23
Number of Volumes
1 vol.
Illustrated
Yes
Dewey Decimal
519.5
Table Of Content
Preface.- 1 Introduction.- 2 Statistical Learning.- 3 Linear Regression.- 4 Classification.- 5 Resampling Methods.- 6 Linear Model Selection and Regularization.- 7 Moving Beyond Linearity.- 8 Tree-Based Methods.- 9 Support Vector Machines.- 10 Deep Learning.- 11 Survival Analysis and Censored Data.- 12 Unsupervised Learning.- 13 Multiple Testing.- Index.
Synopsis
An Introduction to Statistical Learning provides an accessible overview of the field of statistical learning, an essential toolset for making sense of the vast and complex data sets that have emerged in fields ranging from biology to finance to marketing to astrophysics in the past twenty years. This book presents some of the most important modeling and prediction techniques, along with relevant applications. Topics include linear regression, classification, resampling methods, shrinkage approaches, tree-based methods, support vector machines, clustering, deep learning, survival analysis, multiple testing, and more. Color graphics and real-world examples are used to illustrate the methods presented. Since the goal of this textbook is to facilitate the use of these statistical learning techniques by practitioners in science, industry, and other fields, each chapter contains a tutorial on implementing the analyses and methods presented in R, an extremely popular open source statistical software platform. Two of the authors co-wrote The Elements of Statistical Learning (Hastie, Tibshirani and Friedman, 2nd edition 2009), a popular reference book for statistics and machine learning researchers. An Introduction to Statistical Learning covers many of the same topics, but at a level accessible to a much broader audience. This book is targeted at statisticians and non-statisticians alike who wish to use cutting-edge statistical learning techniques to analyze their data. The text assumes only a previous course in linear regression and no knowledge of matrix algebra. This Second Edition features new chapters on deep learning, survival analysis, and multiple testing, as well as expanded treatments of na ve Bayes, generalized linear models, Bayesian additive regression trees, and matrix completion. R code has been updated throughout to ensure compatibility., An Introduction to Statistical Learning provides an accessible overview of the field of statistical learning, an essential toolset for making sense of the vast and complex data sets that have emerged in fields ranging from biology to finance to marketing to astrophysics in the past twenty years. This book presents some of the most important modeling and prediction techniques, along with relevant applications. Topics include linear regression, classification, resampling methods, shrinkage approaches, tree-based methods, support vector machines, clustering, deep learning, survival analysis, multiple testing, and more. Color graphics and real-world examples are used to illustrate the methods presented. Since the goal of this textbook is to facilitate the use of these statistical learning techniques by practitioners in science, industry, and other fields, each chapter contains a tutorial on implementing the analyses and methods presented in R, an extremely popular open source statistical software platform. Two of the authors co-wrote The Elements of Statistical Learning (Hastie, Tibshirani and Friedman, 2nd edition 2009), a popular reference book for statistics and machine learning researchers. An Introduction to Statistical Learning covers many of the same topics, but at a level accessible to a much broader audience. This book is targeted at statisticians and non-statisticians alike who wish to use cutting-edge statistical learning techniques to analyze their data. The text assumes only a previous course in linear regression and no knowledge of matrix algebra. This Second Edition features new chapters on deep learning, survival analysis, and multiple testing, as well as expanded treatments of naïve Bayes, generalized linear models, Bayesian additive regression trees, and matrix completion. R code has been updated throughout to ensure compatibility.
LC Classification Number
QA276-280
Item description from the seller
About this seller
lindseys1907
118 items sold
Registered as a private sellerThereby, consumer rights stemming from EU consumer protection law do not apply. eBay buyer protection still applies to most purchases.
Seller Feedback (41)
- e***m (4)- Feedback left by buyer.More than a year agoVerified purchaseThe product came well packaged and quickly. Thank youCoach Envelope Wallet Signature Canvas Leather (#285117189453)
- h***o (2140)- Feedback left by buyer.More than a year agoVerified purchaseLightening fast shipping- Nice item and as described. ThanksPANDORA Moments Charm Bangle Bracelet With Sparkling Lines Openwork Charm (#284782592957)
- d***h (1013)- Feedback left by buyer.More than a year agoVerified purchaseGreat seller! Thank You.Apple AirPods 2nd Generation with Charging Case - White (#285117182617)
Product ratings and reviews
Most relevant reviews
- 03 Apr, 2024
Wonderful book.
Verified purchase: YesCondition: NewSold by: nkb_24
- 05 Mar, 2025
Very nice book that goes into the comput...
Verified purchase: YesCondition: NewSold by: bookshelf-treasures
More to explore:
- Statistics Adult Learning & University Textbook,
- Statistics Adult Learning & University Books,
- Statistics Paperback Adult Learning & University Books in English,
- Oxford University Press Statistics Adult Learning & University Books,
- Statistics School Textbooks and Study Guides,
- Look and Learn Magazines,
- May Look and Learn Magazines,
- Adult Learning & University Books,
- Adult Learning and University Technology Book,
- Adult Learning and University Chemistry Book